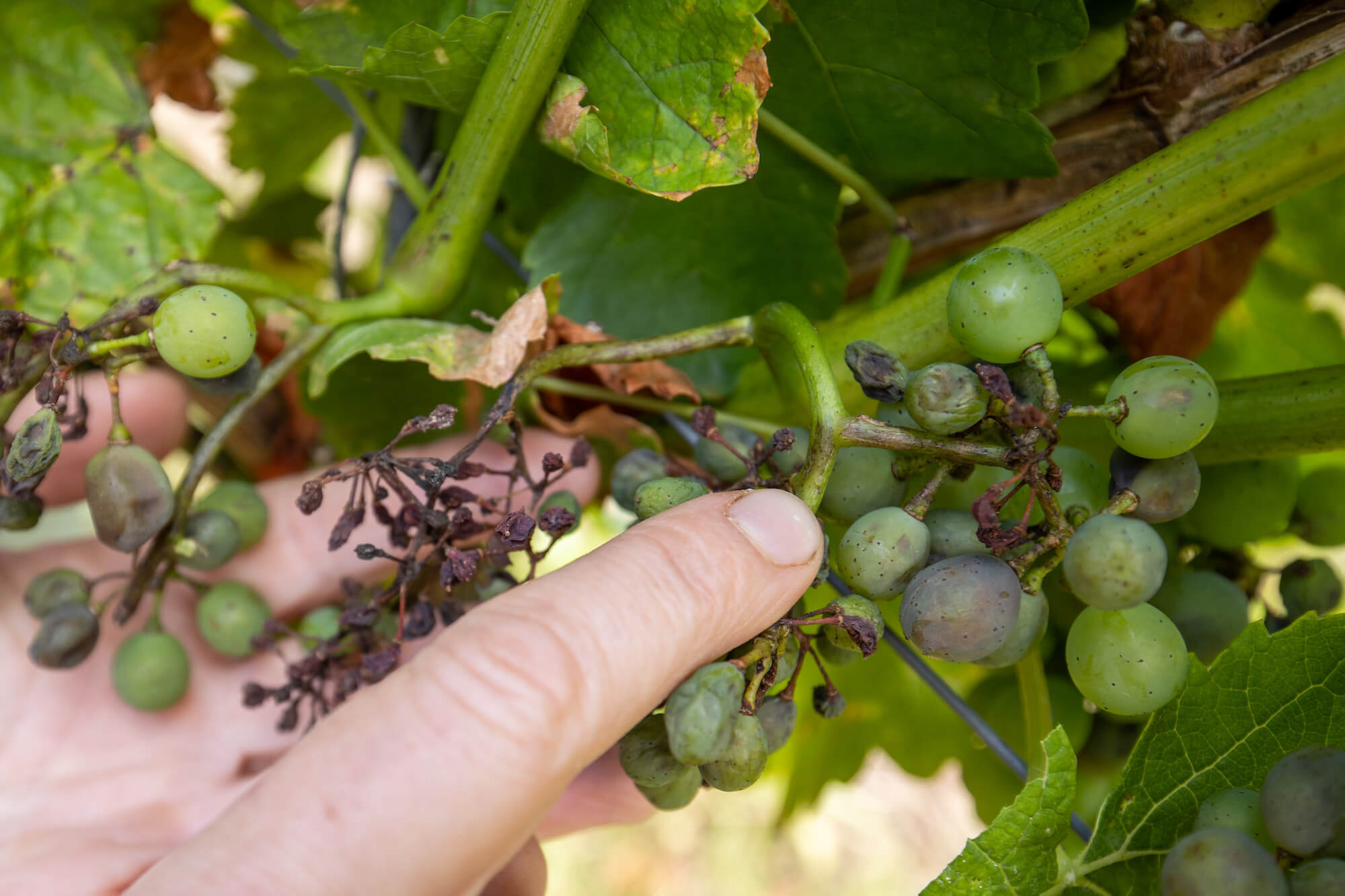
Daniel Karmann/picture alliance via Getty Images
You read that right. Emerging AI technology is helping researchers determine how different cultivars respond to diseases like powdery mildew, with the aim of breeding pathogen-resistant varieties down the line.
It used to take plant pathologist Lance Cadle-Davidson and his team months to assess the severity of fungal infections on grape seedlings they were studying. Now, this exacting process might take a few days at most—and they have robots equipped with facial recognition technology to thank for that.
Advancements in microscopic imaging are helping grape breeders take a closer, more accurate look at how plants react to powdery mildew—a common fungal disease that attacks vineyards late in the growing season and can spoil entire harvests. The pathogen is a costly one to tackle: In California, the country’s biggest producer of grapes by far, researchers estimated that powdery mildew growers spend approximately $239 million per year on powdery mildew management, and accounted for 90 percent of their pesticide use.
Now, powerful imaging robots are helping researchers automate some of the most laborious parts of the grape breeding process, which in turn could significantly reduce the amount of time it takes to develop new, disease-resistant varieties. Down the line, growers are banking on these incoming grape cultivars to save them millions in lost crops and pesticide costs, according to a news release from Cornell University, which hosts the grape research collaborative co-directed by Cadle-Davidson.
“What this has opened up is the capability to be strategic about how we develop new varieties,” said Cadle-Davidson. “Not just arbitrarily picking a resistance gene to cross into cultivated grapes, but to pick the one that’s going to work for the longest time. [The technology] is enabling us to do experiments to figure out which two or which three resistance genes will work best together.”
“What this has opened up is the capability to be strategic about how we develop new varieties. Not just arbitrarily picking a resistance gene to cross into cultivated grapes, but to pick the one that’s going to work for the longest time.”
Cadle-Davidson leads the collaborative’s powdery mildew team, which seeks to discover which grape genes are responsible for expressing resistance to the fungus. (In technical terms, this work is called “phenotyping.”) Solving these fruity mysteries involves hours of meticulous, yet virtually motionless work in front of a microscope: Cadle-Davidson explained that each experiment involves examining hundreds of grape leaf samples for infection on a cell-by-cell basis.
Here’s how it typically goes: The leaf samples in question are first cut into small disks measuring about one centimeter in diameter. Researchers then zoom in on each disk to inspect each cell for infection. Finally, they add up the number of sick cells to estimate the total level of infection. Taken together, this is strenuous work. Imagine taking a magnifying glass to observe every micron on an object half the size of a penny. Now imagine doing that hundreds of times for every experiment. Now imagine doing those experiments six times a year, year after year.
Now imagine if there was a high-resolution imaging robot that could take on the heavy lifting. This kind of technology could significantly shorten the duration of each study, enabling breeders to conduct a lot more research in the same amount of time.
“The methods that we’ve developed over the course of the past 10 years were really slow and required sitting at a microscope for one month or two months to execute,” said Cadle-Davidson. “So that’s where automation and artificial intelligence come in to really speed up those controlled experiments.”
As more data—in this case, grape leaf photos—get fed into the algorithm, the program in turn becomes more proficient at accurately calculating the severity of infections.
In 2019, a colleague of Cadle-Davidson’s named Yu Jiang, an agricultural robotics researcher and assistant professor at Cornell, began to develop a camera with the ability to take extremely close-up photos of the grape leaf samples. Dubbed “Blackbird,” the dedicated technology can blow a one-centimeter leaf disk up to an image with a resolution measuring 5,000 by 8,000 pixels, Jiang explained.
“At that resolution, what you can see is all the microscopic level organs of that leaf disk,” he said. “Specifically, if we inoculate powdery mildew on the grape leaf disk, you will see sporulation of that particular pathogen.”
Already, this eliminates the need for a researcher to hunch over a leaf sample placed under a microscope and manually inspect it cell-by-cell. But Blackbird doesn’t just simplify the work of zooming in on grape leaf samples. It also streamlines the process of quantifying just how infected each sample is. After taking high-resolution photos, Blackbird then feeds them into an algorithm, which calculates the precise level of infection within each leaf sample—based on a fragment-by-fragment analysis of each image. As more data—in this case, grape leaf photos—get fed into the algorithm, the program in turn becomes more proficient at accurately calculating the severity of infections. Think of it as facial recognition technology, but for pathogen-exposed fruit. All of that information then gets routed back to the Cadle-Davidson’s team, where it’s used to help them locate the genes responsible for regulating disease resistance in a given grape cultivar.
“It helps us at a very early stage eliminate the grape vines that are clearly not going to be future cultivars. So we can focus our attention on the elite grapevines.”
That information is necessary to have on hand, because it plays a guiding role in plant breeding. To understand why, it’s helpful to remember how traditional breeding works: Throughout history, growers have attempted to cross-pollinate parent plants with different desirable traits—think flavor, sturdiness, productivity—in order to create hybrids that check all the boxes. Not only is that process time-consuming, it can also be something of a shot in the dark. You may find yourself with a hybrid grape that is both seedless and delicious. Or you might find yourself with one that is neither of those things. Knowing which genes to include gives breeders a lot more certainty about the characteristics that they can expect.
“It helps us at a very early stage eliminate the grape vines that are clearly not going to be future cultivars,” Cadle-Davidson explained. “So we can focus our attention on the elite grapevines.”
Don’t expect elite grapes to hit grocery stores just yet. Cadle-Davidson estimates that disease-resistant varieties that can significantly reduce fungicide use are still about 10 years away. In the meantime, he and Jiang are already exploring whether Blackbird’s imaging technology can be used to help breed varieties of other fruits and vegetables.
“We’re finding that [this technology] works really well for powdery mildews of all crops—we’re using it to understand disease resistance in strawberries and hops,” Cadle-Davidson said. “The science is only going to continue to get better.”